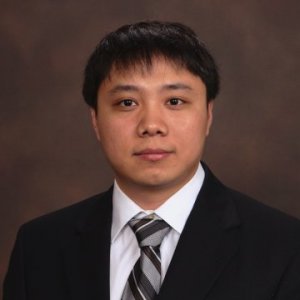
Yonghui Wu
Director/Associate Professor
- Gainesville FL UNITED STATES
- College of Medicine
Yonghui Wu’s research is in computer science and biomedical informatics to develop medical artificial intelligence to solve medical problems
Contact More Open optionsBiography
Dr. Yonghui Wu is an associate professor in the College of Medicine, Department of Health Outcomes & Biomedical Informatics at the University of Florida. He also serves as the director of Natural Language Processing (NLP) at UF Clinical and Translational Science Institute (CTSI) and OneFlorida Clinical Research Consortium. He has worked on various challenging research topics including large language models, patient information extraction, NLP-powered computable phenotyping, disease predictive modeling, and many other artificial intelligence (AI) applications in the medical domain. His work was supported by funding from the National Institutes of Health (NIH), Patient-Centered Outcomes Research Institute (PCORI), and Centers for Disease Control and Prevention (CDC).
Areas of Expertise
Social
Articles
A study of generative large language model for medical research and healthcare
NPJ Digital MedicineCheng Peng, et. al
2023-11-16
This study develops a generative clinical LLM, GatorTronGPT, using 277 billion words of text including (1) 82 billion words of clinical text from 126 clinical departments and approximately 2 million patients at the University of Florida Health and (2) 195 billion words of diverse general English text. We train GatorTronGPT using a GPT-3 architecture with up to 20 billion parameters and evaluate its utility for biomedical natural language processing (NLP) and healthcare text generation.
The role of health system penetration rate in estimating the prevalence of type 1 diabetes in children and adolescents using electronic health records
Journal of the American Medical Informatics AssociationPiaopiao Li, et. al
2023-10-09
Having sufficient population coverage from the electronic health records (EHRs)-connected health system is essential for building a comprehensive EHR-based diabetes surveillance system. This study aimed to establish an EHR-based type 1 diabetes (T1D) surveillance system for children and adolescents across racial and ethnic groups by identifying the minimum population coverage from EHR-connected health systems to accurately estimate T1D prevalence.
Clinical Prediction Models for Hospital-Induced Delirium Using Structured and Unstructured Electronic Health Record Data: Protocol for a Development and Validation Study
JMIR Research ProtocolsSarah E. Ser, et. al
2023-09-11
Hospital-induced delirium is one of the most common and costly iatrogenic conditions, and its incidence is predicted to increase as the population of the United States ages. An academic and clinical interdisciplinary systems approach is needed to reduce the frequency and impact of hospital-induced delirium.
Clinical concept and relation extraction using prompt-based machine reading comprehension
Journal of the American Medical Informatics AssociationCheng Peng, et. al
2023-06-14
To develop a natural language processing system that solves both clinical concept extraction and relation extraction in a unified prompt-based machine reading comprehension (MRC) architecture with good generalizability for cross-institution applications. We formulate both clinical concept extraction and relation extraction using a unified prompt-based MRC architecture and explore state-of-the-art transformer models.
Assess the documentation of cognitive tests and biomarkers in electronic health records via natural language processing for Alzheimer’s disease and related dementias.
International Journal of Medical InformaticsZhaoyi Chen, et. al
2022-12-17
In this work, we aim to (1) assess the documentation of cognitive tests and biomarkers in EHRs that can be used as real-world endpoints, and (2) identify, extract, and harmonize the different commonly used cognitive tests from clinical narratives using natural language processing (NLP) methods into categorical AD/ADRD severity. We developed a rule-based NLP pipeline to extract the cognitive tests and biomarkers from clinical narratives in AD/ADRD patients’ EHRs.